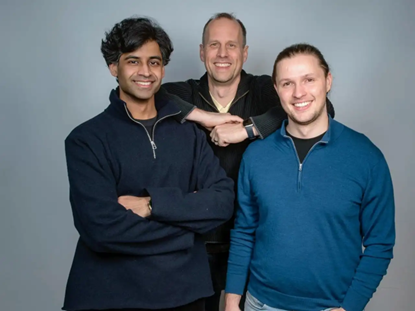
企業(yè)內(nèi)部的許多信息都是以行和列呈現(xiàn)的所謂的“表格數(shù)據(jù)”,。例如報(bào)告中的電子數(shù)據(jù)表、數(shù)據(jù)庫(kù)條目與大量圖表等,。
事實(shí)證明,,由于多個(gè)原因,人工智能模型很難處理表格數(shù)據(jù),。表格中有時(shí)是文本,,有時(shí)是數(shù)字,而且數(shù)字還有不同的計(jì)量單位,,可以說(shuō)是令人困惑的大雜燴,。此外,表格中不同單元格之間的關(guān)系有時(shí)候并不明確。要了解各單元格之間的相互影響,,需要具備專(zhuān)業(yè)知識(shí),。
多年來(lái),機(jī)器學(xué)習(xí)研究人員一直在努力解決表格數(shù)據(jù)的分析問(wèn)題?,F(xiàn)在,,一組研究人員聲稱(chēng)他們找到了一個(gè)優(yōu)雅的解決方案:一個(gè)大型基礎(chǔ)模型。這個(gè)模型類(lèi)似于支持OpenAI的ChatGPT等產(chǎn)品的大語(yǔ)言模型,,但專(zhuān)門(mén)使用表格數(shù)據(jù)進(jìn)行訓(xùn)練,。這個(gè)預(yù)訓(xùn)練模型可以應(yīng)用于任何表格數(shù)據(jù)集,只需幾個(gè)示例,,就能準(zhǔn)確推斷各單元格數(shù)據(jù)之間的關(guān)系,,并且比以往任何機(jī)器學(xué)習(xí)方法都能更好地預(yù)測(cè)缺失數(shù)據(jù)。
弗蘭克·哈特和諾亞·霍爾曼是兩位來(lái)自德國(guó)的計(jì)算機(jī)科學(xué)家,,他們幫助開(kāi)創(chuàng)了這種技術(shù),,并最近在著名的科學(xué)期刊《自然》(Nature)上發(fā)表了一篇論文。他們選擇與有金融從業(yè)經(jīng)驗(yàn)的蘇拉吉·甘比爾合作,,創(chuàng)辦了一家名為Prior Labs的初創(chuàng)公司,,致力于將該技術(shù)商業(yè)化。
近期,,總部位于德國(guó)弗萊堡的Prior Labs宣布已獲得900萬(wàn)歐元(930萬(wàn)美元)種子前融資,。這輪融資由總部位于倫敦的風(fēng)險(xiǎn)投資公司Balderton Capital領(lǐng)投,參投方包括XTX Ventures,、SAP創(chuàng)始人漢斯·沃納-赫克托的赫克托基金(Hector Foundation),、Atlantic Labs和Galion.exe。Hugging Face聯(lián)合創(chuàng)始人兼首席科學(xué)家托馬斯·沃爾夫,、Snyk和Tessl的創(chuàng)始人蓋伊·伯德扎尼,,以及著名的DeepMind研究員艾德·格里芬斯泰特等知名天使投資人也參與了此次融資。
Balderton Capital合伙人詹姆斯·懷斯在解釋為什么決定投資Prior Labs的一份聲明中表示:“表格數(shù)據(jù)是科學(xué)和商業(yè)的支柱,,但顛覆了文本,、圖像和視頻領(lǐng)域的AI革命對(duì)表格數(shù)據(jù)的影響微乎其微——直到現(xiàn)在?!?
Prior Labs在《自然》雜志上發(fā)表的研究報(bào)告中使用的模型被稱(chēng)為T(mén)abular Prior-Fitted Network(簡(jiǎn)稱(chēng) TabPFN),。但 TabPFN的訓(xùn)練僅使用了表格中的數(shù)值數(shù)據(jù),而不是文本數(shù)據(jù),。Prior Labs公司的AI研究員弗蘭克·哈特曾任職于弗萊堡大學(xué)(University of Freiburg)和圖賓根埃利斯研究所(Ellis Institute Tubingen),。他表示,Prior Labs希望將這個(gè)模型變成多模態(tài),,使它既能理解數(shù)字,,也能理解文本。然后該模型將能夠理解列標(biāo)題并進(jìn)行推理,用戶(hù)也可以像使用基于大語(yǔ)言模型的聊天機(jī)器人一樣,,用自然語(yǔ)言提示與AI系統(tǒng)互動(dòng),。
目前的大語(yǔ)言模型,即使是如OpenAI 的o3等更先進(jìn)的推理模型,,雖然可以回答一些關(guān)于表格內(nèi)容的問(wèn)題,,但它們無(wú)法根據(jù)對(duì)表格數(shù)據(jù)的分析做出準(zhǔn)確預(yù)測(cè)。哈特表示:“大語(yǔ)言模型在這方面表現(xiàn)得非常糟糕,。它們?cè)谶@方面的效果遠(yuǎn)不及預(yù)期,,且分析速度緩慢?!苯Y(jié)果,,大多數(shù)需要分析這類(lèi)數(shù)據(jù)的人都使用了舊的統(tǒng)計(jì)方法,這些方法速度快,,但并不總是最準(zhǔn)確的,。
但Prior Labs的TabPFN能夠做出精準(zhǔn)預(yù)測(cè),包括處理所謂的"時(shí)間序列"數(shù)據(jù)——這類(lèi)預(yù)測(cè)基于復(fù)雜模式,,利用歷史數(shù)據(jù)推斷下一個(gè)最可能的數(shù)據(jù)點(diǎn),。根據(jù)Prior Labs團(tuán)隊(duì)1月發(fā)布在非同行評(píng)審研究平臺(tái)arxiv.org上的新論文顯示,TabPFN在時(shí)間序列預(yù)測(cè)方面的表現(xiàn)優(yōu)于現(xiàn)有模型:較同類(lèi)最佳小型AI模型預(yù)測(cè)準(zhǔn)確率提升7.7%,,甚至超越比其大65倍的模型3%,。
時(shí)間序列預(yù)測(cè)在各行各業(yè)應(yīng)用廣泛,尤其是醫(yī)療和金融等領(lǐng)域,。哈特透露:“對(duì)沖基金對(duì)我們青睞有加,。”(事實(shí)上,,一家對(duì)沖基金已成為其首批客戶(hù)(因保密協(xié)議無(wú)法透露名稱(chēng)),,另一家正在概念驗(yàn)證階段的客戶(hù)是軟件巨頭SAP。)
Prior Labs以開(kāi)源形式發(fā)布TabPFN模型,,唯一許可要求是使用者必須公開(kāi)聲明模型來(lái)源,。哈特稱(chēng),該模型下載量已達(dá)約百萬(wàn)次,。與多數(shù)開(kāi)源AI公司類(lèi)似,Prior Labs計(jì)劃的盈利模式聚焦于針對(duì)客戶(hù)的用例定制模型,,并為特定市場(chǎng)開(kāi)發(fā)工具和應(yīng)用,。
Prior Labs并不是唯一致力于突破AI在表格數(shù)據(jù)方面限制的公司。由麻省理工學(xué)院(MIT)數(shù)據(jù)科學(xué)家德瓦弗拉特·沙阿創(chuàng)立的Ikigai Labs和法國(guó)初創(chuàng)公司Neuralk AI等正嘗試將深度學(xué)習(xí)(包括生成式AI)應(yīng)用于表格數(shù)據(jù),,谷歌(Google)和微軟(Microsoft)的研究團(tuán)隊(duì)也在攻克這一難題,。谷歌云的表格數(shù)據(jù)解決方案部分基于A(yíng)utoML技術(shù)(該技術(shù)使用機(jī)器學(xué)習(xí),將創(chuàng)建有效AI模型所需的步驟自動(dòng)化,哈特曾是該領(lǐng)域的先驅(qū)),。
哈特表示,,Prior將持續(xù)升級(jí)模型:重點(diǎn)開(kāi)發(fā)關(guān)系型數(shù)據(jù)庫(kù)支持、增強(qiáng)時(shí)間序列分析能力,,構(gòu)建“因果發(fā)現(xiàn)”功能(識(shí)別表格數(shù)據(jù)間的因果關(guān)系),,并推出可通過(guò)聊天界面回答表格問(wèn)題的交互功能。他表示:“我們將在第一年實(shí)現(xiàn)這些目標(biāo),?!保ㄘ?cái)富中文網(wǎng))
譯者:劉進(jìn)龍
審校:汪皓
企業(yè)內(nèi)部的許多信息都是以行和列呈現(xiàn)的所謂的“表格數(shù)據(jù)”。例如報(bào)告中的電子數(shù)據(jù)表,、數(shù)據(jù)庫(kù)條目與大量圖表等,。
事實(shí)證明,由于多個(gè)原因,,人工智能模型很難處理表格數(shù)據(jù),。表格中有時(shí)是文本,有時(shí)是數(shù)字,,而且數(shù)字還有不同的計(jì)量單位,,可以說(shuō)是令人困惑的大雜燴。此外,,表格中不同單元格之間的關(guān)系有時(shí)候并不明確,。要了解各單元格之間的相互影響,需要具備專(zhuān)業(yè)知識(shí),。
多年來(lái),,機(jī)器學(xué)習(xí)研究人員一直在努力解決表格數(shù)據(jù)的分析問(wèn)題。現(xiàn)在,,一組研究人員聲稱(chēng)他們找到了一個(gè)優(yōu)雅的解決方案:一個(gè)大型基礎(chǔ)模型,。這個(gè)模型類(lèi)似于支持OpenAI的ChatGPT等產(chǎn)品的大語(yǔ)言模型,但專(zhuān)門(mén)使用表格數(shù)據(jù)進(jìn)行訓(xùn)練,。這個(gè)預(yù)訓(xùn)練模型可以應(yīng)用于任何表格數(shù)據(jù)集,,只需幾個(gè)示例,就能準(zhǔn)確推斷各單元格數(shù)據(jù)之間的關(guān)系,,并且比以往任何機(jī)器學(xué)習(xí)方法都能更好地預(yù)測(cè)缺失數(shù)據(jù),。
弗蘭克·哈特和諾亞·霍爾曼是兩位來(lái)自德國(guó)的計(jì)算機(jī)科學(xué)家,他們幫助開(kāi)創(chuàng)了這種技術(shù),,并最近在著名的科學(xué)期刊《自然》(Nature)上發(fā)表了一篇論文,。他們選擇與有金融從業(yè)經(jīng)驗(yàn)的蘇拉吉·甘比爾合作,創(chuàng)辦了一家名為Prior Labs的初創(chuàng)公司,,致力于將該技術(shù)商業(yè)化,。
近期,,總部位于德國(guó)弗萊堡的Prior Labs宣布已獲得900萬(wàn)歐元(930萬(wàn)美元)種子前融資。這輪融資由總部位于倫敦的風(fēng)險(xiǎn)投資公司Balderton Capital領(lǐng)投,,參投方包括XTX Ventures,、SAP創(chuàng)始人漢斯·沃納-赫克托的赫克托基金(Hector Foundation)、Atlantic Labs和Galion.exe,。Hugging Face聯(lián)合創(chuàng)始人兼首席科學(xué)家托馬斯·沃爾夫,、Snyk和Tessl的創(chuàng)始人蓋伊·伯德扎尼,以及著名的DeepMind研究員艾德·格里芬斯泰特等知名天使投資人也參與了此次融資,。
Balderton Capital合伙人詹姆斯·懷斯在解釋為什么決定投資Prior Labs的一份聲明中表示:“表格數(shù)據(jù)是科學(xué)和商業(yè)的支柱,,但顛覆了文本、圖像和視頻領(lǐng)域的AI革命對(duì)表格數(shù)據(jù)的影響微乎其微——直到現(xiàn)在,?!?
Prior Labs在《自然》雜志上發(fā)表的研究報(bào)告中使用的模型被稱(chēng)為T(mén)abular Prior-Fitted Network(簡(jiǎn)稱(chēng) TabPFN)。但 TabPFN的訓(xùn)練僅使用了表格中的數(shù)值數(shù)據(jù),,而不是文本數(shù)據(jù),。Prior Labs公司的AI研究員弗蘭克·哈特曾任職于弗萊堡大學(xué)(University of Freiburg)和圖賓根埃利斯研究所(Ellis Institute Tubingen)。他表示,,Prior Labs希望將這個(gè)模型變成多模態(tài),,使它既能理解數(shù)字,也能理解文本,。然后該模型將能夠理解列標(biāo)題并進(jìn)行推理,,用戶(hù)也可以像使用基于大語(yǔ)言模型的聊天機(jī)器人一樣,用自然語(yǔ)言提示與AI系統(tǒng)互動(dòng),。
目前的大語(yǔ)言模型,,即使是如OpenAI 的o3等更先進(jìn)的推理模型,雖然可以回答一些關(guān)于表格內(nèi)容的問(wèn)題,,但它們無(wú)法根據(jù)對(duì)表格數(shù)據(jù)的分析做出準(zhǔn)確預(yù)測(cè),。哈特表示:“大語(yǔ)言模型在這方面表現(xiàn)得非常糟糕。它們?cè)谶@方面的效果遠(yuǎn)不及預(yù)期,,且分析速度緩慢,。”結(jié)果,,大多數(shù)需要分析這類(lèi)數(shù)據(jù)的人都使用了舊的統(tǒng)計(jì)方法,,這些方法速度快,但并不總是最準(zhǔn)確的,。
但Prior Labs的TabPFN能夠做出精準(zhǔn)預(yù)測(cè),,包括處理所謂的"時(shí)間序列"數(shù)據(jù)——這類(lèi)預(yù)測(cè)基于復(fù)雜模式,利用歷史數(shù)據(jù)推斷下一個(gè)最可能的數(shù)據(jù)點(diǎn),。根據(jù)Prior Labs團(tuán)隊(duì)1月發(fā)布在非同行評(píng)審研究平臺(tái)arxiv.org上的新論文顯示,,TabPFN在時(shí)間序列預(yù)測(cè)方面的表現(xiàn)優(yōu)于現(xiàn)有模型:較同類(lèi)最佳小型AI模型預(yù)測(cè)準(zhǔn)確率提升7.7%,甚至超越比其大65倍的模型3%,。
時(shí)間序列預(yù)測(cè)在各行各業(yè)應(yīng)用廣泛,,尤其是醫(yī)療和金融等領(lǐng)域。哈特透露:“對(duì)沖基金對(duì)我們青睞有加,?!保ㄊ聦?shí)上,一家對(duì)沖基金已成為其首批客戶(hù)(因保密協(xié)議無(wú)法透露名稱(chēng)),,另一家正在概念驗(yàn)證階段的客戶(hù)是軟件巨頭SAP,。)
Prior Labs以開(kāi)源形式發(fā)布TabPFN模型,唯一許可要求是使用者必須公開(kāi)聲明模型來(lái)源,。哈特稱(chēng),,該模型下載量已達(dá)約百萬(wàn)次。與多數(shù)開(kāi)源AI公司類(lèi)似,,Prior Labs計(jì)劃的盈利模式聚焦于針對(duì)客戶(hù)的用例定制模型,,并為特定市場(chǎng)開(kāi)發(fā)工具和應(yīng)用。
Prior Labs并不是唯一致力于突破AI在表格數(shù)據(jù)方面限制的公司,。由麻省理工學(xué)院(MIT)數(shù)據(jù)科學(xué)家德瓦弗拉特·沙阿創(chuàng)立的Ikigai Labs和法國(guó)初創(chuàng)公司Neuralk AI等正嘗試將深度學(xué)習(xí)(包括生成式AI)應(yīng)用于表格數(shù)據(jù),,谷歌(Google)和微軟(Microsoft)的研究團(tuán)隊(duì)也在攻克這一難題。谷歌云的表格數(shù)據(jù)解決方案部分基于A(yíng)utoML技術(shù)(該技術(shù)使用機(jī)器學(xué)習(xí),,將創(chuàng)建有效AI模型所需的步驟自動(dòng)化,,哈特曾是該領(lǐng)域的先驅(qū))。
哈特表示,,Prior將持續(xù)升級(jí)模型:重點(diǎn)開(kāi)發(fā)關(guān)系型數(shù)據(jù)庫(kù)支持,、增強(qiáng)時(shí)間序列分析能力,構(gòu)建“因果發(fā)現(xiàn)”功能(識(shí)別表格數(shù)據(jù)間的因果關(guān)系),,并推出可通過(guò)聊天界面回答表格問(wèn)題的交互功能,。他表示:“我們將在第一年實(shí)現(xiàn)這些目標(biāo)?!保ㄘ?cái)富中文網(wǎng))
譯者:劉進(jìn)龍
審校:汪皓
A lot of information inside companies is what’s known as “tabular data,” or data that is presented in rows and columns. Think spreadsheets and database entries and lots of figures in reports.
Well, it turns out that artificial intelligence models have difficulty working with tabular data, for several reasons. It’s often a confusing jumble—sometimes text and sometimes numbers, as well as numbers in different units of measurement. What’s more, the relationship between different cells in the table is sometimes unclear. Knowing which cells influence which other cells in a table often requires domain expertise.
For years, machine learning researchers have been trying to crack this tabular data problem. Now, a group of researchers has found what they claim is an elegant solution: A large foundation model—similar to the large language models that underpin products like OpenAI’s ChatGPT—but specifically trained on tabular data. This pre-trained model can then be applied to any tabular data set, and with just a few examples, make accurate inferences about the relationship between data in various cells and also predict missing data better than any prior machine learning method.
Frank Hutter and Noah Hollman, two Germany-based computer scientists who helped pioneer this technique and recently published a paper on it in the prestigious scientific journal Nature, have teamed with Sauraj Gambhir, who has experience in finance, on a startup called Prior Labs dedicated to commercializing this technology.
Today Prior Labs, which is based in Freiburg, Germany, announced it has received 9 million euros ($9.3 million) in pre-seed funding. The round is led by London-based venture capital firm Balderton Capital along with XTX Ventures, SAP founder Hans Werner-Hector’s Hector Foundation, Atlantic Labs, and Galion.exe. A number of prominent angel investors, including Hugging Face cofounder and chief scientist Thomas Wolf, Guy Podjarny, who founded Snyk and Tessl, and Ed Grefenstette, a well-known DeepMind researcher, also participated in the funding.
“Tabular data is the backbone of science and business, yet the AI revolution transforming text, images and video has had only a marginal impact on tabular data–until now,” James Wise, a partner at Balderton Capital, said in a statement, explaining why the firm decided to invest in Prior Labs.
The model Prior Labs used for its Nature study is called a Tabular Prior-Fitted Network (TabPFN for short.) But TabPFN is trained only on the numerical data in tables, not the text. Hutter, a well-known AI researcher formerly at the University of Freiburg and the Ellis Institute Tubingen, said Prior Labs wants to take this model and make it multimodal, so that it can understand both numbers and text. Then the model will be able to understand column headings and reason about them, and users will be able to interact with the AI system using natural language prompts, just like an LLM-based chatbot.
Today’s LLM’s, even the more advanced reasoning models, such as OpenAI’s o3 model, can answer some questions about what a table says, but they can’t make accurate predictions based on an analysis of the data in the table. “LLMs are just horrible at that,” Hutter said. “It’s like, it’s nowhere close. It’s not only that, it’s also super slow.” As a result, most people who needed to analyze this kind of data used older statistical methods that were fast, but not always the most accurate.
But Prior Labs’ TabPFN can make accurate predictions, including on what are called time series, where past data is used to predict the next most likely data point based on complex patterns. In a new paper the Prior Labs team published in January on the non-peer reviewed research repository arxiv.org, the team found that TabPFN outperformed existing time series prediction models. It beat the best previous small AI model for such predictions by 7.7% and beat a model that is 65 times larger than TabPFN by 3%.
Time series prediction has many applications across industries, but especially in medical and financial domains. “Hedge funds love us,” Hutter said. (One of Prior Labs’ initial customers is, in fact, a hedge fund, but Hutter said he was contractually barred from saying which one. Another initial customer with which Hutter is doing a proof of concept is software giant SAP.)
Prior Labs is offering TabPFN as an open source model—with the only license requirement being that if people use the model, they must publicly say so. So far, it has been downloaded about one million times, according to Hutter. Like most open source AI companies, Prior Labs plans to make money by working with specific customers to help them tailor the models to their use case and also by building tools and applications for specific market segments.
Prior Labs is not the only company working to crack AI’s limits when it comes to tabular data. Startups Ikigai Labs, which was founded by MIT data scientist Devarat Shah, and French startup Neuralk AI are among others working on applying deep learning methods, including generative AI, to tabular data. Researchers at Google and Microsoft have also been working on this problem. Google Cloud’s tabular data solutions are built in part on AutoML, a process that uses machine learning to automate the steps needed to create effective AI models, an area that Hutter helped pioneer.
Hutter said Prior intends to keep improving its models, working more on relational databases, time series, and building the ability to do what is called “causal discovery”—where a user asks which data points in a table have a causal relationship with other data in the table. Then there’s the chat feature that will let users ask questions of the tables using a chat-like interface. “All of this we will build in the first year,” he said.