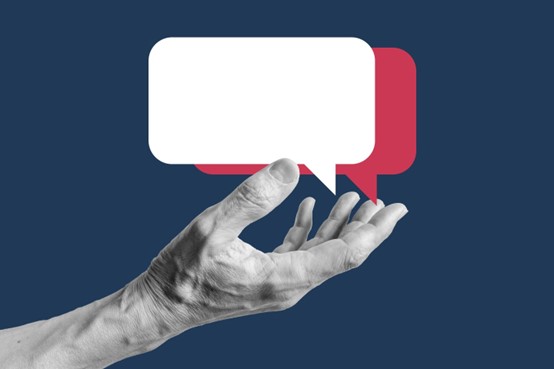
公司之間在人工智能應(yīng)用方面的競爭格局正在發(fā)生變化:它們不再僅僅爭相采用這一技術(shù),,而更加關(guān)注如何有效地運(yùn)用這些強(qiáng)大的工具。隨著企業(yè)逐漸認(rèn)識到,,不明確的提示語,,即指示人工智能執(zhí)行特定任務(wù)的指令,以及非專業(yè)模型的使用,,會導(dǎo)致不準(zhǔn)確的結(jié)果和低效率,,因此它們的態(tài)度開始發(fā)生改變。
這樣的例子不勝枚舉,。強(qiáng)生(Johnson & Johnson)等公司正在創(chuàng)建提示語數(shù)據(jù)庫,,供員工使用,以提高人工智能輸出結(jié)果的質(zhì)量,。星巴克(Starbucks)等其他公司則更進(jìn)一步,,正在創(chuàng)建內(nèi)部模型。
需要強(qiáng)調(diào)的是,,企業(yè)在使用大型語言模型如ChatGPT等時(shí),,應(yīng)提供明確的提示語,例如“總結(jié)這篇故事”等簡單的指令,。這種明確的提示對于有效應(yīng)用人工智能至關(guān)重要,。
斯坦福大學(xué)(Stanford University)計(jì)算機(jī)科學(xué)與語言學(xué)教授、斯坦福人工智能實(shí)驗(yàn)室(Stanford Artificial Intelligence Laboratory)主任克里斯托弗·曼寧對《財(cái)富》雜志表示:“提示語可以分為兩個(gè)部分,。第一部分需要準(zhǔn)確描述你希望完成的任務(wù),,然后需要進(jìn)行不斷的調(diào)整,,因?yàn)槿藗儠芸彀l(fā)現(xiàn)某些提示語的效果更佳。研究表明,,那些簡單,、友好的指令,比如‘務(wù)必仔細(xì)考慮’等,,通常能夠產(chǎn)生良好的效果,。”
提示語數(shù)據(jù)庫可以是開啟人工智能對話的一個(gè)話術(shù)集,,就像強(qiáng)生公司內(nèi)部使用的數(shù)據(jù)庫一樣,,旨在降低員工使用其內(nèi)部生成式人工智能聊天機(jī)器人時(shí)的困難。
強(qiáng)生發(fā)言人表示:“我們使用[我們的聊天機(jī)器人]上傳內(nèi)部文件,,生成摘要或提出特定問題,。我們創(chuàng)建了一個(gè)由啟發(fā)性話題組成的提示語數(shù)據(jù)庫,以幫助員工探索與不同業(yè)務(wù)領(lǐng)域有關(guān)的潛在使用案例,?!?/font>
一些提示語的設(shè)計(jì)旨在降低“幻覺”風(fēng)險(xiǎn),或以盡可能高效的方式生成答案,。所謂的“幻覺”風(fēng)險(xiǎn)指的是人工智能頻繁生成聽起來合理但實(shí)際并不準(zhǔn)確的陳述,。
創(chuàng)意機(jī)構(gòu)GUT的首席情報(bào)官兼合伙人克里斯蒂安·皮埃爾對《財(cái)富》雜志表示:“我們根據(jù)使用案例或預(yù)期結(jié)果,創(chuàng)建了不同提示語數(shù)據(jù)庫,。 [我們的]策略師和數(shù)據(jù)分析師共用一個(gè)數(shù)據(jù)庫和一個(gè)“關(guān)鍵詞參考手冊”,,其中列出的關(guān)鍵詞可以明顯改變輸出結(jié)果。例如,,我們知道,,只要添加‘以布爾查詢的方式回答’,ChatGPT就會編寫布爾查詢語句,,這些語句可用于我們的社交傾聽工具,。”
通常情況下,,導(dǎo)致不盡如人意的結(jié)果出現(xiàn)的原因之一是相關(guān)數(shù)據(jù)集中缺乏必要的知識,。例如,如果一個(gè)提示語問及為什么約翰被車撞到,,大型語言模型可能會嘗試提供答案,,盡管它之前并不了解與約翰或事故有關(guān)的任何信息。
曼寧教授表示:“如果有事實(shí)作為參考,,這些模型將依據(jù)這些事實(shí)來生成答案,。但如果沒有相關(guān)事實(shí)可依,[它可能會]在沒有事實(shí)依據(jù)的情況下盡量編寫看似合理的答案?!?/font>
因此,,要編寫完善的提示語,需要提供豐富的背景信息,,仔細(xì)思考關(guān)鍵詞,,并確切描述所期望的回應(yīng)形式。那些自豪于創(chuàng)作出完美提示語的人通常自稱為"提示語工程師",。
更進(jìn)一步
很可惜,,大多數(shù)經(jīng)過優(yōu)化的提示語,可能無法滿足大公司的需求,。
安永(Ernst & Young)全球咨詢數(shù)據(jù)與人工智能負(fù)責(zé)人比阿特麗斯·桑斯·賽茲對《財(cái)富》雜志表示:“這些大語言模型的訓(xùn)練基于一般化數(shù)據(jù),。而我們正在嘗試引入最優(yōu)秀的人才,例如稅務(wù)專業(yè)人士,,真正對這些模型進(jìn)行調(diào)整,、維護(hù)和反復(fù)操練?!?/font>
安永創(chuàng)建了一個(gè)內(nèi)部人工智能平臺EY.ai,。微軟(Microsoft)為該公司提供了盡早使用Azure OpenAI的權(quán)限,,用于創(chuàng)建一個(gè)安全,、專業(yè)的系統(tǒng)。這幫助安永提高了系統(tǒng)運(yùn)行速度,,保護(hù)了敏感數(shù)據(jù),,而且最重要的是,使安永能夠根據(jù)預(yù)期結(jié)果調(diào)整模型,。
曼寧教授解釋說:“假設(shè)你需要執(zhí)行某項(xiàng)任務(wù),,比如審閱保險(xiǎn)索賠并起草相關(guān)處理方案和理由等,如果你有大量以往的業(yè)務(wù)實(shí)例可供參考,,那么你可以通過微調(diào)模型,,使其特別擅長處理這種任務(wù)?!?/font>
對模型的微調(diào)由具備機(jī)器學(xué)習(xí)經(jīng)驗(yàn)的人負(fù)責(zé),,而不是由提示語工程師進(jìn)行調(diào)整。在這個(gè)階段,,公司可以決定刪除不必要的內(nèi)容,,以精簡數(shù)據(jù)集,例如寫俳句的能力,,或者將模型固定為提供特定的功能,。
安永還創(chuàng)建了一個(gè)嵌入數(shù)據(jù)庫,提高了系統(tǒng)的專業(yè)化程度。
賽茲表示:“[嵌入]可以理解為輸入到模型中的附加數(shù)據(jù)集,。我們可以整合稅務(wù)知識,、國家法規(guī)甚至行業(yè)知識,將所有信息串聯(lián)起來,?!?/font>
通過將這些額外的數(shù)據(jù)集添加到模型中,可以使模型更加適應(yīng)其預(yù)期的用途,。公司發(fā)現(xiàn),,最有效地利用人工智能的方法之一是創(chuàng)建一個(gè)受控的數(shù)據(jù)集,將其嵌入到數(shù)據(jù)庫中,,并使用自定義提示語進(jìn)行查詢,。
賽茲解釋稱:“通常情況下,目前我們能做的是基于安永多年來積累的集體智慧,,而不是個(gè)別稅務(wù)團(tuán)隊(duì)的專業(yè)經(jīng)驗(yàn),,對客戶進(jìn)行評估。而且我們不止在一個(gè)地區(qū)這樣做,,而是在全球多個(gè)地區(qū)采用這種做法,。”
賽茲認(rèn)為,,通過微調(diào)內(nèi)部模型和整合數(shù)據(jù)庫,,將人工智能模型個(gè)性化,將成為未來公司使用人工智能的關(guān)鍵,。她還預(yù)測,,隨著人工智能的智能水平不斷提高,提示語的重要性將逐漸降低,。
然而,,曼寧教授認(rèn)為未來有多種可能。未來,,既會有專業(yè)系統(tǒng)用于處理大工作量的任務(wù),,也會有廣義模型,需要根據(jù)經(jīng)過設(shè)計(jì)的提示語處理非常規(guī)任務(wù),,例如編寫招聘廣告,。
曼寧教授對《財(cái)富》雜志表示:“現(xiàn)在你可以把這些重要的任務(wù)交給ChatGPT來完成。我認(rèn)為,,許多公司可以成功地培養(yǎng)出專門人才,,學(xué)習(xí)并精通編寫提示語,從而讓ChatGPT生成令人滿意的結(jié)果,?!保ㄘ?cái)富中文網(wǎng))
翻譯:劉進(jìn)龍
審校:汪皓
攝影:BORIS ZHITKOV —— 蓋蒂圖片社
公司之間在人工智能應(yīng)用方面的競爭格局正在發(fā)生變化:它們不再僅僅爭相采用這一技術(shù),而更加關(guān)注如何有效地運(yùn)用這些強(qiáng)大的工具。隨著企業(yè)逐漸認(rèn)識到,,不明確的提示語,,即指示人工智能執(zhí)行特定任務(wù)的指令,以及非專業(yè)模型的使用,,會導(dǎo)致不準(zhǔn)確的結(jié)果和低效率,,因此它們的態(tài)度開始發(fā)生改變。
這樣的例子不勝枚舉,。強(qiáng)生(Johnson & Johnson)等公司正在創(chuàng)建提示語數(shù)據(jù)庫,,供員工使用,以提高人工智能輸出結(jié)果的質(zhì)量,。星巴克(Starbucks)等其他公司則更進(jìn)一步,,正在創(chuàng)建內(nèi)部模型。
需要強(qiáng)調(diào)的是,,企業(yè)在使用大型語言模型如ChatGPT等時(shí),,應(yīng)提供明確的提示語,例如“總結(jié)這篇故事”等簡單的指令,。這種明確的提示對于有效應(yīng)用人工智能至關(guān)重要,。
斯坦福大學(xué)(Stanford University)計(jì)算機(jī)科學(xué)與語言學(xué)教授、斯坦福人工智能實(shí)驗(yàn)室(Stanford Artificial Intelligence Laboratory)主任克里斯托弗·曼寧對《財(cái)富》雜志表示:“提示語可以分為兩個(gè)部分,。第一部分需要準(zhǔn)確描述你希望完成的任務(wù),,然后需要進(jìn)行不斷的調(diào)整,因?yàn)槿藗儠芸彀l(fā)現(xiàn)某些提示語的效果更佳,。研究表明,,那些簡單,、友好的指令,,比如‘務(wù)必仔細(xì)考慮’等,通常能夠產(chǎn)生良好的效果,?!?/p>
提示語數(shù)據(jù)庫可以是開啟人工智能對話的一個(gè)話術(shù)集,就像強(qiáng)生公司內(nèi)部使用的數(shù)據(jù)庫一樣,,旨在降低員工使用其內(nèi)部生成式人工智能聊天機(jī)器人時(shí)的困難,。
強(qiáng)生發(fā)言人表示:“我們使用[我們的聊天機(jī)器人]上傳內(nèi)部文件,生成摘要或提出特定問題,。我們創(chuàng)建了一個(gè)由啟發(fā)性話題組成的提示語數(shù)據(jù)庫,,以幫助員工探索與不同業(yè)務(wù)領(lǐng)域有關(guān)的潛在使用案例?!?/p>
一些提示語的設(shè)計(jì)旨在降低“幻覺”風(fēng)險(xiǎn),,或以盡可能高效的方式生成答案。所謂的“幻覺”風(fēng)險(xiǎn)指的是人工智能頻繁生成聽起來合理但實(shí)際并不準(zhǔn)確的陳述。
創(chuàng)意機(jī)構(gòu)GUT的首席情報(bào)官兼合伙人克里斯蒂安·皮埃爾對《財(cái)富》雜志表示:“我們根據(jù)使用案例或預(yù)期結(jié)果,,創(chuàng)建了不同提示語數(shù)據(jù)庫,。 [我們的]策略師和數(shù)據(jù)分析師共用一個(gè)數(shù)據(jù)庫和一個(gè)“關(guān)鍵詞參考手冊”,其中列出的關(guān)鍵詞可以明顯改變輸出結(jié)果,。例如,,我們知道,只要添加‘以布爾查詢的方式回答’,,ChatGPT就會編寫布爾查詢語句,,這些語句可用于我們的社交傾聽工具?!?/p>
通常情況下,,導(dǎo)致不盡如人意的結(jié)果出現(xiàn)的原因之一是相關(guān)數(shù)據(jù)集中缺乏必要的知識。例如,,如果一個(gè)提示語問及為什么約翰被車撞到,,大型語言模型可能會嘗試提供答案,盡管它之前并不了解與約翰或事故有關(guān)的任何信息,。
曼寧教授表示:“如果有事實(shí)作為參考,,這些模型將依據(jù)這些事實(shí)來生成答案。但如果沒有相關(guān)事實(shí)可依,,[它可能會]在沒有事實(shí)依據(jù)的情況下盡量編寫看似合理的答案,。”
因此,,要編寫完善的提示語,,需要提供豐富的背景信息,仔細(xì)思考關(guān)鍵詞,,并確切描述所期望的回應(yīng)形式,。那些自豪于創(chuàng)作出完美提示語的人通常自稱為"提示語工程師"。
更進(jìn)一步
很可惜,,大多數(shù)經(jīng)過優(yōu)化的提示語,,可能無法滿足大公司的需求。
安永(Ernst & Young)全球咨詢數(shù)據(jù)與人工智能負(fù)責(zé)人比阿特麗斯·桑斯·賽茲對《財(cái)富》雜志表示:“這些大語言模型的訓(xùn)練基于一般化數(shù)據(jù),。而我們正在嘗試引入最優(yōu)秀的人才,,例如稅務(wù)專業(yè)人士,真正對這些模型進(jìn)行調(diào)整,、維護(hù)和反復(fù)操練,。”
安永創(chuàng)建了一個(gè)內(nèi)部人工智能平臺EY.ai,。微軟(Microsoft)為該公司提供了盡早使用Azure OpenAI的權(quán)限,,用于創(chuàng)建一個(gè)安全,、專業(yè)的系統(tǒng)。這幫助安永提高了系統(tǒng)運(yùn)行速度,,保護(hù)了敏感數(shù)據(jù),,而且最重要的是,使安永能夠根據(jù)預(yù)期結(jié)果調(diào)整模型,。
曼寧教授解釋說:“假設(shè)你需要執(zhí)行某項(xiàng)任務(wù),,比如審閱保險(xiǎn)索賠并起草相關(guān)處理方案和理由等,如果你有大量以往的業(yè)務(wù)實(shí)例可供參考,,那么你可以通過微調(diào)模型,,使其特別擅長處理這種任務(wù)?!?/p>
對模型的微調(diào)由具備機(jī)器學(xué)習(xí)經(jīng)驗(yàn)的人負(fù)責(zé),,而不是由提示語工程師進(jìn)行調(diào)整。在這個(gè)階段,,公司可以決定刪除不必要的內(nèi)容,,以精簡數(shù)據(jù)集,例如寫俳句的能力,,或者將模型固定為提供特定的功能,。
安永還創(chuàng)建了一個(gè)嵌入數(shù)據(jù)庫,提高了系統(tǒng)的專業(yè)化程度,。
賽茲表示:“[嵌入]可以理解為輸入到模型中的附加數(shù)據(jù)集,。我們可以整合稅務(wù)知識、國家法規(guī)甚至行業(yè)知識,,將所有信息串聯(lián)起來,。”
通過將這些額外的數(shù)據(jù)集添加到模型中,,可以使模型更加適應(yīng)其預(yù)期的用途,。公司發(fā)現(xiàn),最有效地利用人工智能的方法之一是創(chuàng)建一個(gè)受控的數(shù)據(jù)集,,將其嵌入到數(shù)據(jù)庫中,,并使用自定義提示語進(jìn)行查詢。
賽茲解釋稱:“通常情況下,,目前我們能做的是基于安永多年來積累的集體智慧,而不是個(gè)別稅務(wù)團(tuán)隊(duì)的專業(yè)經(jīng)驗(yàn),,對客戶進(jìn)行評估,。而且我們不止在一個(gè)地區(qū)這樣做,而是在全球多個(gè)地區(qū)采用這種做法,?!?/p>
賽茲認(rèn)為,,通過微調(diào)內(nèi)部模型和整合數(shù)據(jù)庫,將人工智能模型個(gè)性化,,將成為未來公司使用人工智能的關(guān)鍵,。她還預(yù)測,隨著人工智能的智能水平不斷提高,,提示語的重要性將逐漸降低,。
然而,曼寧教授認(rèn)為未來有多種可能,。未來,,既會有專業(yè)系統(tǒng)用于處理大工作量的任務(wù),也會有廣義模型,,需要根據(jù)經(jīng)過設(shè)計(jì)的提示語處理非常規(guī)任務(wù),,例如編寫招聘廣告。
曼寧教授對《財(cái)富》雜志表示:“現(xiàn)在你可以把這些重要的任務(wù)交給ChatGPT來完成,。我認(rèn)為,,許多公司可以成功地培養(yǎng)出專門人才,學(xué)習(xí)并精通編寫提示語,,從而讓ChatGPT生成令人滿意的結(jié)果,。”(財(cái)富中文網(wǎng))
翻譯:劉進(jìn)龍
審校:汪皓
BORIS ZHITKOV—GETTY IMAGES
The race among companies to adapt AI has evolved: Instead of simply striving to be first, firms have turned their attention to learning how to deploy these powerful tools effectively. This development comes as companies discover that poorly crafted prompts—the set of instructions used to tell AI to perform a given task—and the use of unspecialized models are spawning inaccuracies and inefficiencies.
There are many examples of this evolution. Firms like Johnson & Johnson are creating libraries of prompts to share among staff to improve the quality of AI output. Other companies, including Starbucks, are taking things further by creating in-house models.
For context, it’s helpful to know that using a so-called large language model, like ChatGPT, requires entering a prompt for the AI that can be as simple as “summarize this story.”
“There are two parts of prompting. One part is just to give a good description of what you want done,” Christopher Manning, professor of computer science and linguistics at Stanford University and director of the Stanford Artificial Intelligence Laboratory, told Fortune. “After that, there’s a lot of fiddling that goes on because people quickly find that some prompts work better than others. It turns out that giving grandmotherly instructions like ‘make sure you think carefully about it’ actually tend to do good.”
Prompt libraries can be as simple as a collection of conversation starters like the library Johnson & Johnson’s uses in order to reduce friction for employees who use its internal generative AI chatbot.
“We’re using [our chatbot] to upload internal documents and create summaries or ask ad hoc questions,” a spokesperson for Johnson & Johnson said. “We created a prompt library with thought-starters to help employees explore potential use cases relevant to different areas of the business.”
Meanwhile other prompts aim to minimize the risk of hallucination—the term for the frequent occurrence of AI producing facts that sound plausible but aren’t true—or to format answers in the most efficient way possible.
“We have different prompting libraries depending on the use case or expected output,” Christian Pierre, Chief Intelligence Officer & Partner at creative agency GUT, told Fortune. “[Our] strategists and data analysts share a library and a ‘keyword cheat sheet’ with specific keywords that can drastically change the output. For instance, we know that just by adding ‘Provide it as a boolean query,’ ChatGPT will write boolean queries that we can use in our social listening tools.”
Often undesired outputs are the result of the absence of knowledge in the relevant data set. For example, a language model will likely supply an answer to a prompt asking why John got hit by a car—even if it has no information about John or the accident in the first place.
“The tendency of all of these models is that if there are facts, they will use them.” Professor Manning said, “And if there aren’t facts, [it will] write plausible ones with no basis in truth.”
Thus crafting the perfect prompt requires providing an intense level of context, the tweaking of keywords, and a precise description of the desired form. Those who pride themselves on creating these call themselves prompt engineers.
Taking it even further
Unfortunately, even the most optimized prompt can fall short of what big companies are looking for.
“These large language models are very generically trained,” Beatriz Sanz Sáiz, global consulting data and AI leader at Ernst & Young, told Fortune. “What we are trying to achieve is really bring in the best, let’s say tax professionals, to really fine-tune, retain, and retrain.”
Ernst & Young has created an in-house AI platform called EY.ai. Microsoft provided the firm early access to Azure OpenAI, in order to build a secure and specialized system. This has helped increase the speed of the system and protect sensitive data and, most importantly, provided EY with the ability to adjust the model to fit its desired outcomes.
“If there’s one task that you want to do—like reading insurance claims, writing out what we’re doing with them, and what the reason for it was—and if you’ve got a fair number of examples of that from your past business,” Professor Manning explained, “you can then fine-tune the model to be especially good at that.”
Fine-tuning is done by someone with machine learning experience, rather than a prompt engineer. At this stage, the company may decide to shrink the dataset by removing unnecessary stuff, like the ability to write haiku, to fixate the model on a specific function.
Ernst & Young has specialized its system further by creating a library of embeddings.
“Think of [embeddings] as additional data sets that you put into the model,” Sáiz said. “We can connect all the dots by bringing together the tax knowledge, the country regulation, maybe also the sector knowledge.”
By plugging-in these additional datasets, the model becomes hyper-specific to its purpose. Companies are finding the best AI recipe entails building on a controlled dataset, injecting it with a library of embeddings, and querying with customized prompts.
“Typically now what we’ll be able to do is assess clients, not on the expertise of an individual tax team but on the collective knowledge that EY has created for years,” Sáiz explained. “And not just in one jurisdiction, but globally across multiple jurisdictions.”
Sáiz believes that the personalization of AI models through finely tuned in-house models and embedding libraries will be crucial to the future of companies using AI. She also predicts that the importance of prompts will decrease as AI gets more intelligent.
However, Professor Manning believes the future will be mixed. While specialized systems will exist for high volume tasks there is also room for generalized models that require engineered prompts for irregular tasks, such as writing a job advertisement.
“Those are great tasks you can give to ChatGPT these days,” Professor Manning told Fortune. “I think a huge space of companies can very successfully have someone who learns a bit and gets perfectly decent at writing prompts and getting great results out of ChatGPT.”